Effectiveness of traditional Chinese medicine revealed and predicted by network medicine framework
- zuobiaoyuan
- Nov 12, 2023
- 4 min read
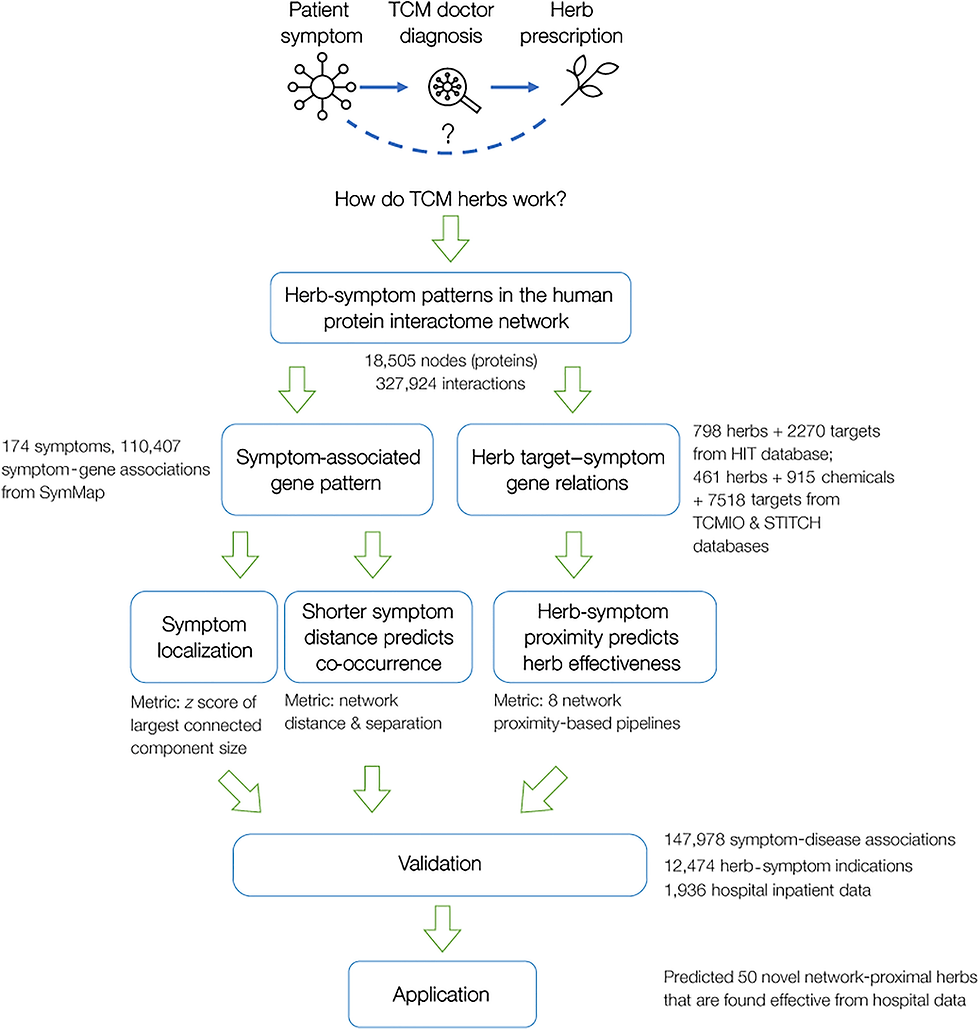
This manuscript is published on Science Advances, a highly recognized journal.
Understanding the therapeutic effects of traditional and natural medicine can lead to drug discoveries that reshape world welfare. For example, aspirin (acetylsalicylic acid) is extracted from willow bark, a traditional medicine practice since thousands of years ago. More recently, the 2015 Nobel Prize was given to the discovery of the malaria-treating artemisinin, extracted from qinghao (Artemisia annua), an herb used in traditional Chinese medicine (TCM). As a famous practice of natural medicine, TCM is a personalized and holistic approach to treating diseases using natural medical products tailored to a patient’s symptoms, offering a rich pool of therapeutic candidates. However, although clinical data and studies of single herbs/prescriptions showed that certain TCM herbal treatments are effective, the general mechanistic principle of how TCM selects herbs to treat diseases remains unknown. Two major challenges exist in understanding the mechanistic root of TCM: (i) The lack of scientific foundation in classic TCM theory obstructs the understanding of TCM from a modern biomedical perspective; (ii) the complexity of herbs’ chemical composition and the often-unknown therapeutic protein targets of the chemicals makes conventional brute-force herb/chemical screening infeasible. Therefore, to understand and exploit the therapeutic mechanisms of TCM, it is necessary to establish a framework that can connect TCM knowledge to modern biomedical science and can handle the complexity of herb composition and target data.
An in silico strategy to understand the therapeutic effect of a natural product is to leverage the multiple protein targets of its composing chemicals via network pharmacology (8) and network medicine. Network pharmacology emphasizes the “network target, multi-components” paradigm that complements conventional research’s focus on single targets. This approach has helped researchers identify herbal chemicals with therapeutic potentials, better understand mechanisms of action, and discover drugs. However, existing TCM network pharmacology studies are limited to single herbs or single prescriptions, unable to explain the totality of TCM herb-disease relations. Moreover, many network pharmacology approaches only consider herbs/drugs that target disease genes directly, unable to account for network effects, e.g., when the impact of perturbing a target emerges further downstream and is mediated by protein interactions. Here, we propose avenues to overcome these limitations and improve our understanding of the therapeutic effects of natural products.
Network medicine leverages the human protein-protein interactome (PPI) to reveal disease and drug patterns . The PPI is a network consisting of nodes that are proteins that link to each other by physical (binding) interactions. Network medicine showed that disease-associated proteins tend to form locally clustered modules in the PPI, and shorter network distance between two disease modules is indicative of their comorbidity; moreover, drug efficacy can be predicted by leveraging the network relation between drug targets and disease modules, leading to the development of drug-repurposing methodologies.
In the current study, a network medicine framework that theorizes the scientific basis of TCM as the topological relationship between symptom-associated proteins and herb targets on the protein interactome is developed. By focusing on symptoms rather than diseases, the approach aligns with the TCM practice of diagnosing and treating patients based on their symptom phenotypes. It is discovered that proteins associated with a symptom tend to cluster into a local PPI module, and the network proximity between an herb’s targets and a symptom module is indicative of the herb’s effectiveness in treating the symptom. The network medicine framework with empirical data and hospital patient data is validated and its potential in identifying herb discovery/repurposing opportunities is highlighted.
This work opens up multiple follow-up directions for future work. For example, prioritizing effective herb-symptom pairs can lead to phenotype-based herb/chemical screening, identifying potential treatments against specific disease phenotypes. Furthermore, this framework might be further investigated to reveal interactome patterns that prioritize therapeutic chemicals from an effective herb’s chemical composition. Next, given that the herb-chemical-target data and symptom-gene association data are still incomplete (e.g., the authors lack the chemical dose dependence in herb-chemical mapping), improving and refining these data can enhance our framework’s ability to capture herb-disease treatment relations. The authors also used a dataset focusing on patients with liver cirrhosis, with a subset of the data available for statistical significance in bioinformatic analysis. Extending the size of the patient data could offer better support for our framework. Another future direction is to explore alternative methods/metrics to tackle the diversity/complexity challenge in the herb-chemical-target relations, better capturing herb-symptom relations. Here, the authors used distance-based metrics to define the network relations; developing more complicated methods may increase the predictive power; or consensus algorithms may help balance the results of multiple prediction pipelines. For example, the authors also tested network embedding-based methods, finding that they can also capture herb-symptom proximity (text S1 and fig. S5). Last, the PPI pattern of TCM prescriptions, or herb combinations, is another under-explored direction. Recent works showed that co-prescribed herbs tend to be close in the protein interactome. According to the classic TCM concept, each herb in a prescription has a specific effect, which is often complementary to the effects of other co-prescribed herbs. This established framework to study herb-symptom relations may improve the understanding of how a combination of herbs works against a given symptom or symptom set.
Reference:
Xiao Gan, Zixin Shu, Xinyan Wang, Dengying Yan, Jun Li, Shany Ofaim, Réka Albert, Xiaodong Li, Baoyan Liu, Xuezhong Zhou, Albert-László Barabási. Network medicine framework reveals generic herb-symptom effectiveness of traditional Chinese medicine. Sci Adv. 2023 Oct 27;9(43):eadh0215. doi: 10.1126/sciadv.adh0215.
Commenti